Artificial Intelligence: Building Trust for the Technology of the Future
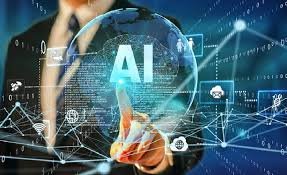
Introduction to the Promise of Artificial Intelligence
Artificial Intelligence (AI) has swiftly transitioned from a futuristic concept to an integral part of our daily lives. The current state of AI showcases a technology that is not only rapidly evolving but also permeating various industries with profound implications. From healthcare to finance, AI is being harnessed to enhance efficiency, precision, and innovation. In healthcare, AI-driven diagnostic tools are enabling early detection of diseases, personalized treatment plans, and improved patient outcomes. Meanwhile, in finance, AI algorithms are optimizing trading strategies, detecting fraudulent activities, and providing personalized financial advice to individuals and businesses alike.
The excitement surrounding AI advancements is palpable, as it promises to revolutionize how we live and work. AI-powered virtual assistants, such as Siri and Alexa, are becoming household staples, simplifying tasks and providing information at our fingertips. Autonomous vehicles are poised to transform transportation, potentially reducing accidents and easing traffic congestion. Moreover, AI’s role in environmental sustainability is gaining traction, with applications in climate modeling, energy management, and wildlife conservation.
However, the rapid integration of AI also introduces a spectrum of uncertainties and challenges. While AI holds immense potential for societal benefits, it is crucial to acknowledge its dual nature as a double-edged sword. Concerns over data privacy, algorithmic bias, and the potential displacement of jobs due to automation are pressing issues that need to be addressed. The ethical implications of AI decision-making processes and the transparency of these systems are areas that require continuous scrutiny and regulation. As we stand on the brink of an AI-driven future, it is imperative to build trust in this technology by ensuring that its development and deployment are guided by principles of fairness, accountability, and inclusivity.
Challenges in Designing, Developing, and Validating AI
The lifecycle of artificial intelligence development encompasses several intricate challenges, beginning with the initial design of algorithms. One of the foremost challenges is ensuring that AI algorithms are efficient while also being unbiased and ethical. Designing algorithms that can process vast amounts of data without perpetuating existing biases requires a nuanced understanding of both technical and social dimensions. For instance, a facial recognition system that misidentifies individuals based on racial or gender attributes exemplifies the critical need for fairness in AI design.
Development of AI systems further complicates this landscape. Real-world variability presents a significant hurdle; AI must be capable of adapting to an array of unpredictable and dynamic environments. A self-driving car, for example, must interpret and react to countless scenarios, from sudden changes in weather to unexpected pedestrian behavior. Ensuring that AI systems can reliably navigate such complexities demands rigorous testing and continuous learning capabilities.
Validation processes are equally vital yet fraught with difficulty. The safety and reliability of AI systems hinge on extensive validation protocols that often require simulating numerous scenarios to assess performance comprehensively. For instance, in healthcare, AI algorithms used for diagnostic purposes must undergo stringent validation to confirm their accuracy and safety across diverse patient populations. A failure to adequately validate these systems can lead to misdiagnoses, with potentially severe consequences.
Several well-documented cases highlight the current shortcomings and failures in AI development. One notable example is the 2018 incident involving Uber’s self-driving car, which fatally struck a pedestrian. The incident underscored the deficiencies in the vehicle’s object detection and decision-making algorithms, emphasizing the need for more robust validation and ethical considerations in AI design.
In summary, the journey from designing to validating AI systems is laden with challenges. Addressing these complexities is crucial to building trust in artificial intelligence as a reliable and ethical technology of the future.
Ensuring Reliability and Safety in AI Modules
The credibility and safety of artificial intelligence (AI) systems are paramount as they become increasingly integrated into diverse sectors. To reinforce the reliability of AI technologies, robust testing frameworks are essential. These testing protocols simulate a wide array of real-world scenarios to identify potential vulnerabilities and ensure that AI systems can handle unexpected inputs without malfunctioning. The development of these frameworks involves extensive collaboration between AI developers and domain experts to tailor tests that are both comprehensive and relevant.
In addition to rigorous testing, the incorporation of fail-safes is a critical strategy in enhancing the safety of AI modules. Fail-safes are designed to prevent catastrophic failures by automatically reverting the system to a safe state in the event of a malfunction. This mechanism is particularly crucial in high-stakes environments such as healthcare, autonomous driving, and industrial automation, where the consequences of AI errors can be severe. By embedding these safety nets within AI systems, developers can significantly mitigate risks and bolster trust in their technology.
Another fundamental aspect of ensuring reliability and safety is the transparency and explainability of AI decision-making processes. Transparency involves making the internal workings and logic of AI systems accessible and understandable to stakeholders, including users, developers, and regulators. Explainability goes a step further by providing clear and comprehensible explanations for the decisions made by AI. This clarity is vital for diagnosing errors, improving system performance, and fostering user trust. Techniques such as model interpretability, which elucidate how AI algorithms arrive at specific decisions, are increasingly being adopted to meet these needs.
Furthermore, the establishment of performance standards by regulatory and standard-setting bodies is instrumental in shaping the development of reliable AI technologies. Organizations such as the International Organization for Standardization (ISO) and the Institute of Electrical and Electronics Engineers (IEEE) are actively working on creating guidelines and benchmarks that AI systems must adhere to. These standards aim to ensure consistency, safety, and quality across different AI applications, thereby promoting a uniform level of reliability and fostering public confidence in AI innovations.
Future Directions and Expectations for AI Trustworthiness
The future of Artificial Intelligence (AI) hinges significantly on its ability to earn and maintain public trust. As AI technologies continue to evolve, anticipated advancements in AI research are expected to enhance the transparency and reliability of these systems. Researchers are increasingly focusing on explainable AI (XAI) to provide clear insights into how AI models make decisions, which is crucial for building user confidence. Additionally, the integration of robust ethical frameworks and fairness algorithms aims to mitigate biases and ensure equitable outcomes for all users.
Interdisciplinary collaboration is another vital component in addressing AI-related issues. By bringing together experts from fields such as computer science, ethics, law, and social sciences, we can develop comprehensive solutions that consider diverse perspectives and the complex nature of AI challenges. This collaborative approach is essential for creating policies and regulations that balance innovation with societal values and norms, thereby fostering a more trustworthy AI ecosystem.
Continuous monitoring and improvement are imperative for maintaining AI trustworthiness. This involves regular audits and assessments of AI systems to identify and rectify potential risks or shortcomings. Feedback mechanisms should be established to gather user input and adapt AI models accordingly. Moreover, fostering a culture of transparency within AI development organizations can help in openly addressing any issues that arise, further reinforcing public trust.
A balanced approach is essential as we navigate the future of AI. While recognizing the immense potential of AI to transform various sectors, it is equally important to remain vigilant about the ethical implications and potential risks associated with its deployment. By prioritizing transparency, interdisciplinary collaboration, and continuous improvement, we can build a trustworthy AI landscape that benefits society as a whole.